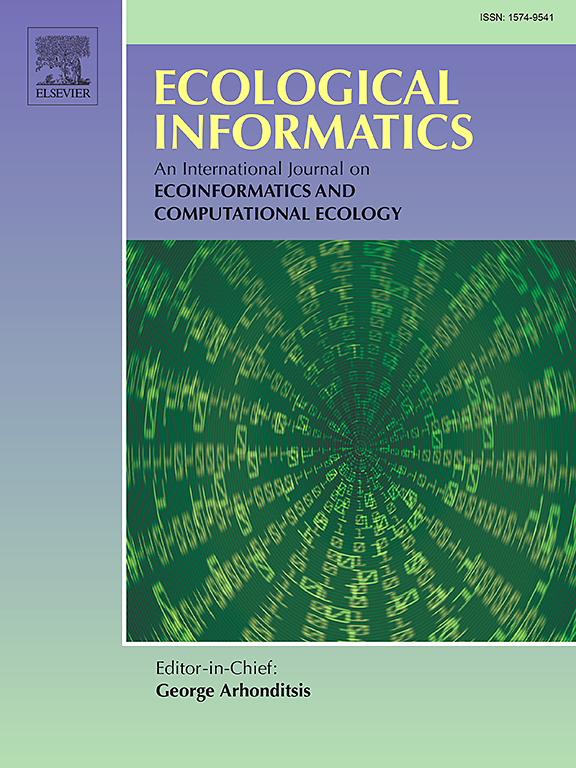
Machine-learning aiding sustainable Indian Ocean tuna purse seine fishery
Among the various challenges facing tropical tuna purse seine fleet are the need to reduce fuel consumption and carbon footprint, as well as minimising bycatch of vulnerable species. Tools designed for forecasting optimum tuna fishing grounds can contribute to adapting to changes in fish distribution due to climate change, by identifying the location of new suitable fishing grounds, and thus reducing the search time. While information about the high probability to find vulnerable species could result in a bycatch reduction. The present study aims at contributing to a more sustainable and cleaner fishing, i.e. catching the same amount of target tuna with less fuel consumption/emissions and lower bycatch. To achieve this, tropical tuna catches as target species, and silky shark accidental catches as bycatch species have been modelled by machine learning models in the Indian Ocean using as inputs historical catch data of these fleets and environmental data. The resulting models show an accuracy of 0.718 and 0.728 for the SKJ and YFT, being the absences (TPR = 0.996 for SKJ and 0.993 for YFT, respectively) better predicted than the high or low catches. In the case of the BET, which is not the main target species of this fleet, the accuracy is lower than that of the previous species. Regarding the silky shark, the presence/absence model provides an accuracy of 0.842. Even though the model’s performance has room for improvement, the present work lays the foundations of a process for forecasting fishing grounds avoiding vulnerable species, by only using as input data forecast environmental data provided in near real time by earth observation programs. In the future these models can be improved as more input data and knowledge about the main environmental conditions influencing these species becomes available.
Read the original work here:
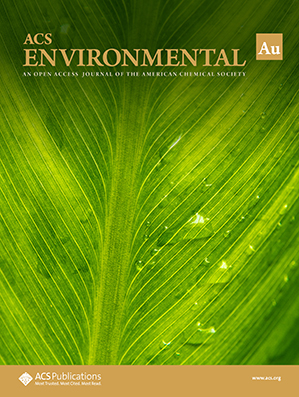
SusTunTech project publishes its digitalization approach to convert fishing vessels in oceanographic data gathering platforms.
Fishing vessels need to adapt to and mitigate climate changes, but solutions development requires better information about environment and vessel operations. Even if ships generate large amounts of potentially useful data, there is a large variety in sources and formats. This lack of standardisation makes identification and use of key data challenging and hinders its use in improving operational performance and vessel design. The work described in this paper aims to provide cost-effective tools for systematic data acquisition for fishing vessels, supporting digitalisation of the fishing vessels operation and performance monitoring. This digitalization is needed to facilitate the reduction of emissions as a critical environmental problem and industry costs critical for the industry sustainability. The resulting monitoring system interfaces onboard systems and sensors, process the data and makes it available in a shared onboard data space. From this data space, 209 signals are recorded at different frequencies and uploaded to onshore servers for post-processing. The collected data describe both ship operation, onboard energy system and the surrounding environment. Nine of the oceanographic variables have been preselected to be potentially useful for public scientific repositories such as Copernicus and EMODnet. The data are also used for fuel prediction models, species distribution models and route optimization models.
Read the original work here:
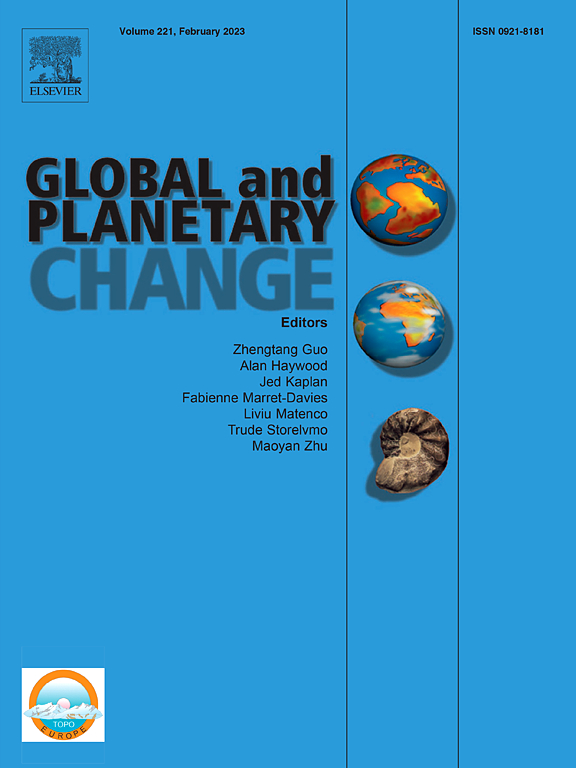
Implications for the global tuna fishing industry of climate change-driven alterations in productivity and body sizes
The AZTI technology centre has led an international study that has concluded that, by 2050, the tuna populations analysed will see a reduction in their average size. In this work, the effects of climate change and fishing on seven tuna and swordfish species distributed and commercially exploited on a global scale have been evaluated under a series of climate change scenarios (RCP 2.6 and 8.5) and fishing scenarios (from no fishing to 1.5 times fishing mortality (F) at Maximum Sustainable Yield, MSY) based on two Earth System Models (IPSL and MEDUSA). It is estimated that five species, Atlantic and southern bluefin tuna, swordfish, bigeye tuna and albacore, will decrease in biomass and size at different rates. On average, body size is expected to decrease by up to 15% by 2050. In this sense, the fishing industry can adapt to climate change by increasing the value of fish through sustainability certifications and reducing fuel consumption and time at sea through increased digitalisation. Reducing fuel consumption would also be an additional climate change mitigation measure, as it would reduce CO2 emissions.
Read the original work here:
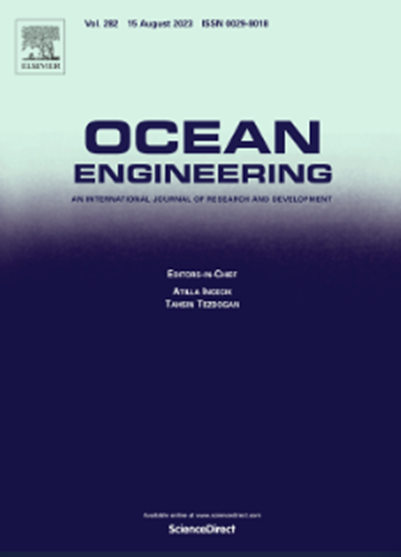
Predicting ship fuel consumption using a combination of metocean and on-board data
Fuel Oil Consumption (FOC) accounts for a significant proportion of a vessel’s operating costs. The cost of fuel for a fishing vessel operation may often go up to 50% or more. Accurate forecasting FOC in voyage planning stage is essential for route optimization decision support system with the objective of fuel-saving, which is difficult because the future state of the vessel and its power and machinery systems for fuel modelling are not available during route planning stage. Moreover, the state of the environment conditions and its impact on vessel performance should be considered. In this paper, machine learning approaches were applied to predict FOC from plannable in-situ variables and modelled speed through water. The latter is estimated from speed over ground and environmental variables in this work, whose prediction is also critical for decision support systems to avoid collisions. By applying the proposed methodology, the final selected Random Forest models can achieve high mean accuracies (over 92%) in predicting fuel consumption on unseen future data.
Read the original work here:
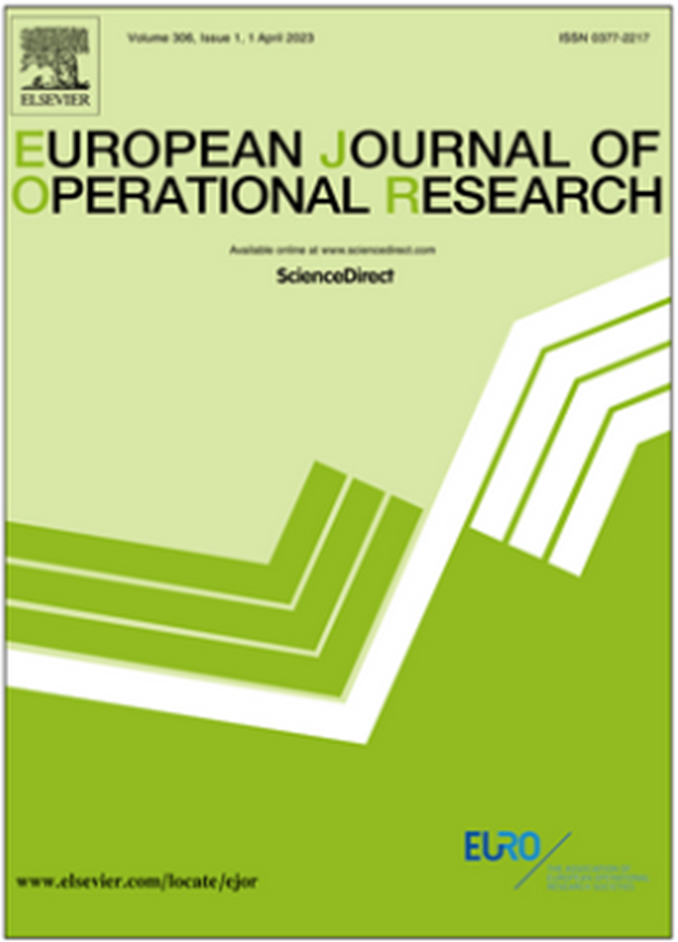
A fishing route optimization decision support system: The case of the tuna purse seiner
Fisheries face challenges in improving efficiency and reducing their emission footprint and operating costs. Decision support systems offer an opportunity to tackle such challenges. This study focuses on the dynamic fishing routing problem using real fishing data. The work solved as a real dynamic problem with route updates every time after a fishing event. The results obtained by this approach were compared with historical fishing trips, where a potential saving in fuel consumption and time at sea of around 57% and 33%, respectively were shown. The algorithm shows that a better selection of fishing grounds together with considerations about weather conditions can help industry to mitigate and adapt to climate change while decreasing one of their main operational costs.
Read the original work here:
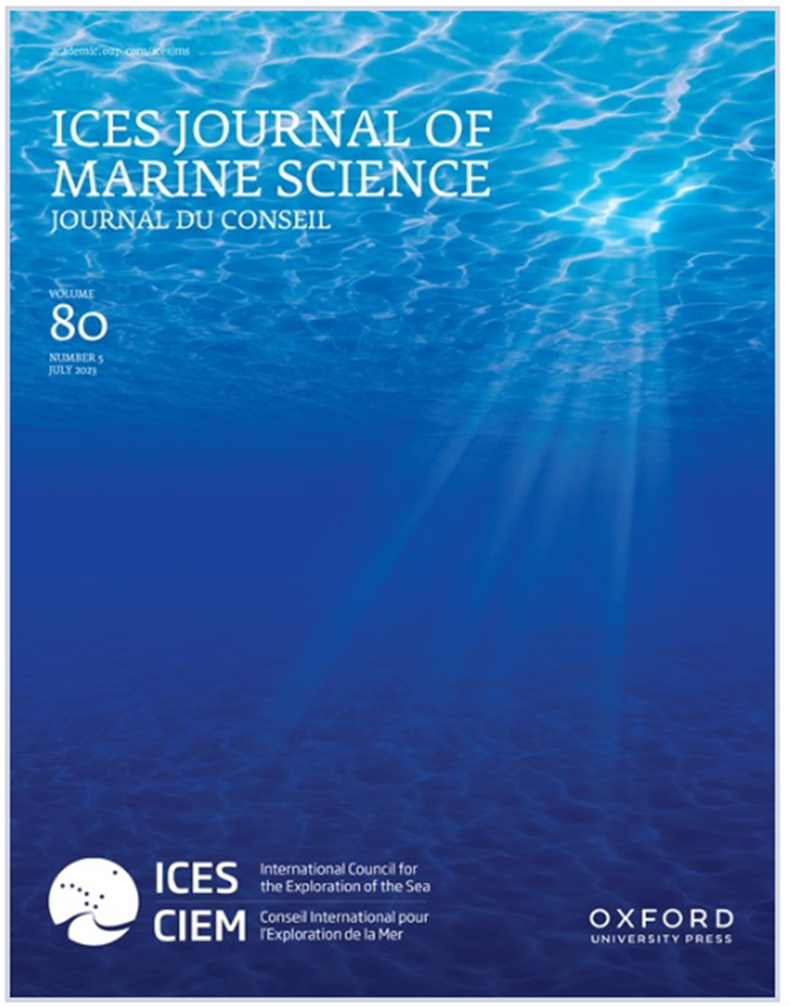
Machine learning in marine ecology: an overview of techniques and applications
Machine learning (ML) covers a large set of algorithms that can be trained to identify patterns in data. Thanks to increase in the amount of data and computing power available, it has become pervasive across scientific disciplines. This work provides a quick primer on ML techniques and vocabulary. For various data types (images, optical spectra, acoustics, omics, geolocations, biogeochemical profiles, and satellite imagery), we present a historical perspective on applications that pro v ed influential and can serve as templates for new work or represent the diversity of approaches. The work highlights the potential use of ML for understanding fishers behaviour and fishing pressure to improve the sustainability of their operations.
Read the original work here:
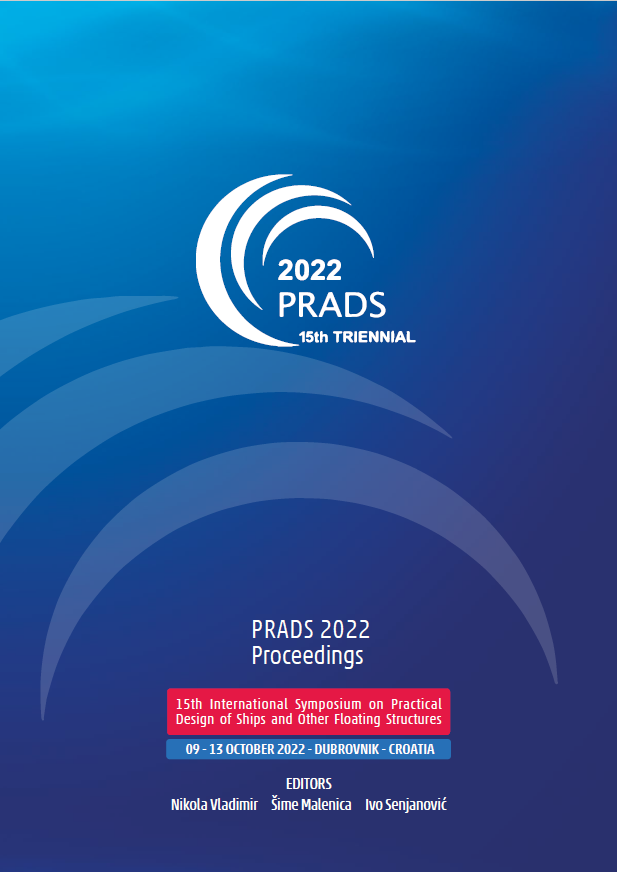
SusTunTech paper at PRADS conference online
PRADS (International Symposium on Practical Design of Ships and Other Floating Structures) is a traditional series of triennial symposia, aiming at an international exchange of new knowledge and achievements with regard to the design, research and development of ships and other floating structures. Dr. Yi Zhou was selected to give a talk and publish a paper about the fuel consumption forecasting models being developed in SusTunTech within a collaboration of Newcastle University, University of Basque Country and AZTI.
Read the original work here:
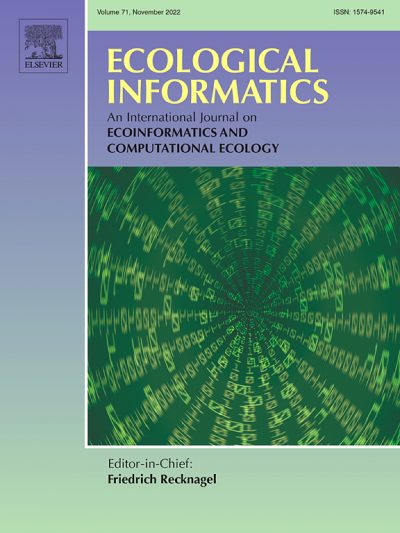
SusTunTech project identifies and measures tropical tuna species through electronic monitoring
An article written by members of the SusTunTech project, and led by AZTI, on the comparison of monitoring by human observers and electronic monitoring with automatic processing on board tuna vessels reveals that 1) fishery monitoring programs are essential for the effective management of marine resources; 2) monitoring by human observers implies a high cost; 3) electronic monitoring performs effective monitoring and is an alternative or complement to human observers; 4) With this system, fish are first segmented individually using a deep neural network (Mask R-CNN); 5) Those segments are passed through another deep neural network (ResNet50V2) to classify them by species and estimate their size distribution; 6) Fish classification with an accuracy of more than 70% is achieved with this method; 7) Size distribution estimates are aligned with official port measurements.
Read the original work here:
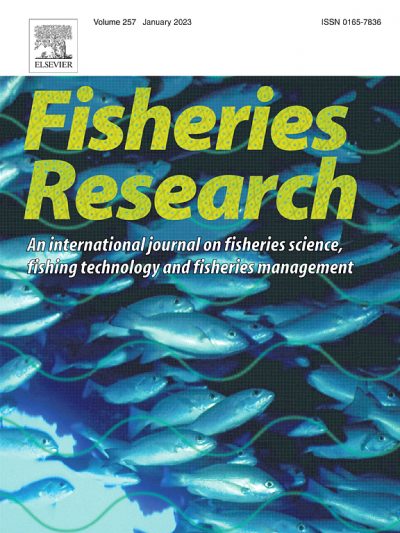
SusTunTech project analyse fishing behaviour to reduce fuel consumption
An article written by members of SusTunTech project, and led by AZTI, about comparing fish aggregating device (FAD) and free-swimming school (FSC) fishing strategies in tropical tuna purse seiner reveal that: 1) Cruising is the most dominant activity in a fishing trip of these fleets; 2) Main engine consumes 75% of the total fuel consumption; 3) FAD fishing often in more fuel intensive due to longer trips aiming at the FADs with higher biomass without considering distance; 4) FAD fishing presents higher success than FSC; 5) both FAD and FSC fishing are more energy efficient than longline, trolling, or pole and line fisheries for Atlantic tuna, but similar or slightly less efficient than Maldivian pole and liners.
Read the original work here:
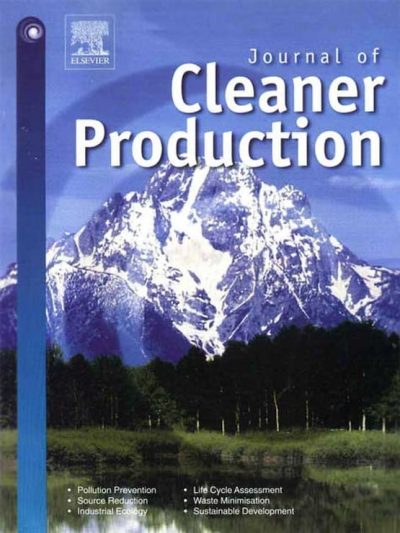
Towards a framework for fishing route optimization decision support systems: Review of the state-of-the-art and challenges
Route optimization methods offer an opportunity to the fisheries industry to enhance their efficiency, sustainability, and safety. However, the use of route optimization Decision Support Systems (DSS), which have been widely used in the shipping industry, is limited in the case of fisheries. In the first part, this work describes the fishing routing problems, reviews the state-of-the-art methods applied in the shipping industry, and introduces a general framework for fishing route optimization decision support systems (FRODSS). In the second part, we highlight the existing gap for the application of DSS in fisheries, and how to develop a FRODSS considering the different types of fishing fleets. Finally, and using the diverse Basque fishing fleet as a case study, we conclude that fishing fleets can be summarized into four main groups whose fishing routes could be optimized in a similar way. This characterization is based on their similarities, such us the target species, fishing gear, and the type and distance to the fishing grounds. These four groups are: (i) small-scale coastal fleet; (ii) large-scale pelagic fleet; (iii) large-scale demersal fleet; and (iv) the distant-water fleet. Distant-water vessels are currently the fleet that can more easily benefit from FRODSS, and they are used as an example here. However, the rest of the fleets could also benefit through adequate adaptation to their operation characteristics, driven by their specific fishing gear and target species.
Read the original work here: